Case Studies
Top stories across all Categories and Topics
Fraud detection for Polish Ministry of Health
Fraud detection for Polish Ministry...
GovTech Polska is using the competition formula to involve tech startups in solving state-scale technological challenges through Artificial Intelligence and Data Science. The central entity is the public sector, which reports challenges and looks for modern ways to solve them but the indirect beneficiaries are of course citizens.
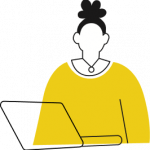
LogicAI Team22 Nov 2022
Price optimization
Price optimization...
Price is one of the most vulnerable parts of any online venture. Every store is trying hard to find that sweet spot where the price is both profitable and emphasizes the value of the product. Instead of going the hard way, Frisco, the Polish market e-grocery giant, decided to approach this in a smart way by leveraging the power of machine learning.
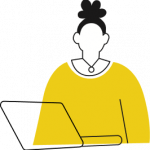
LogicAI Team22 Nov 2022
Predicting defaults for small and medium businesses
Predicting defaults for small and m...
Predicting defaults and risk of small and medium businesses is a challenge that every financial institution has. Alior Bank wanted to enrich its...
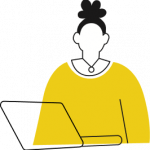
LogicAI Team21 Nov 2022
Matching product to category tree automation
Matching product to category tree a...
For a large e-commerce business, it's crucial to match its products with the merchants' offers as fast as possible. With the COVID-19 and rapid increase in online shopping, it came to a boiling point. Our client, an online platform of diverse goods, wanted to automate the onboarding of new merchants.
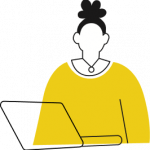
LogicAI Team20 Nov 2022
Predicting student progress, optimizing classes schedules
Predicting student progress, optimi...
Predicting any kind of human behaviour is a difficult task, predicting student progress in online/offline courses is no exception. Our client, an international language school, wanted to improve scheduling of their offline classes by predicting students’ progress in the online part of the course.
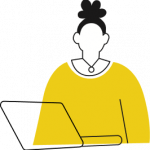
LogicAI Team16 Nov 2022
An award winning & privacy oriented Recommendation Engine
An award winning & privacy ori...
Recommendation system in AVT.pl. Sklep.avt.pl is an e-commerce company that sells electronic appliancesand components for professional engineers and people who want to learn how to assemble electronic appliances. When we started cooperating we suggested additional placements for recommendations.
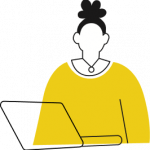
LogicAI Team15 Nov 2022
Fiber optic sensing data analysis application
Fiber optic sensing data analysis a...
Distributed fiber optic sensing, in particular Distributed Temperature Sensing (DTS) and Distributed Acoustic Sensing (DAS), is a technology that allows in-well monitoring e.g. for leakage detection. In order to make sense to a reservoir engineer, measurements need to be processed and visualized.
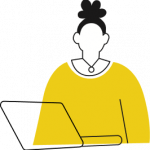
LogicAI Team13 Nov 2022
Investment and savings optimization for Limitless
Investment and savings optimization...
Fintech products are inseparable from the AI industry. AI makes fintech safe, accurate, fair and effective. It is crucial for financial apps to have solid data management and algorithms.
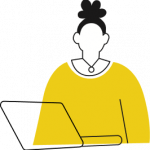
LogicAI Team12 Nov 2022
Kaggle Days Paris Competition
Kaggle Days Paris Competition...
For our second and more chic event, Kaggle Days in Paris, we selected the competition to be about forecasting the sales of the next three months based on the first seven days of sales after launch.
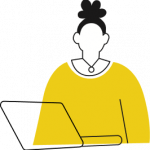
LogicAI Team11 Nov 2022
Inline inspection
Inline inspection...
Inline Inspection involves evaluating pipelines by using intelligent devices to search for internal and external damage. Such inspections are very costly and are carried out every couple of years.
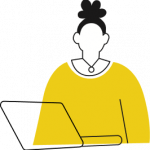
LogicAI Team10 Nov 2022
Digitalization introduction – Training and mentorship for Sanofi
Digitalization introduction –...
During the workshop, participants were presented with basics of AI - the proper definition, use of AI in everyday’s tools, different types of artificial intelligence and common misconceptions about it. Then we talked about Big data, AL, ML and deep learning.
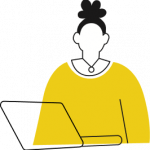
LogicAI Team09 Nov 2022
Building Data Science Community for UAE
Building Data Science Community for...
Better World Hackathon was the first step towards building a strong AI community in UAE and also a part of International Exhibition for National Security and Resilience in Abu Dhabi, UAE.
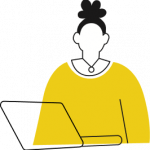
LogicAI Team08 Nov 2022